Unleashing Generative AI in Drug Discovery: A Revolutionary Approach
Have you ever wondered how new life-saving medicines are discovered and developed? It’s a long and extremely expensive process that can take over a decade! But what if we could speed things up using powerful artificial intelligence (AI) technology? That’s where generative AI comes in. In this article we are going to drill down What is the role of Generative AI in Drug Discovery ?
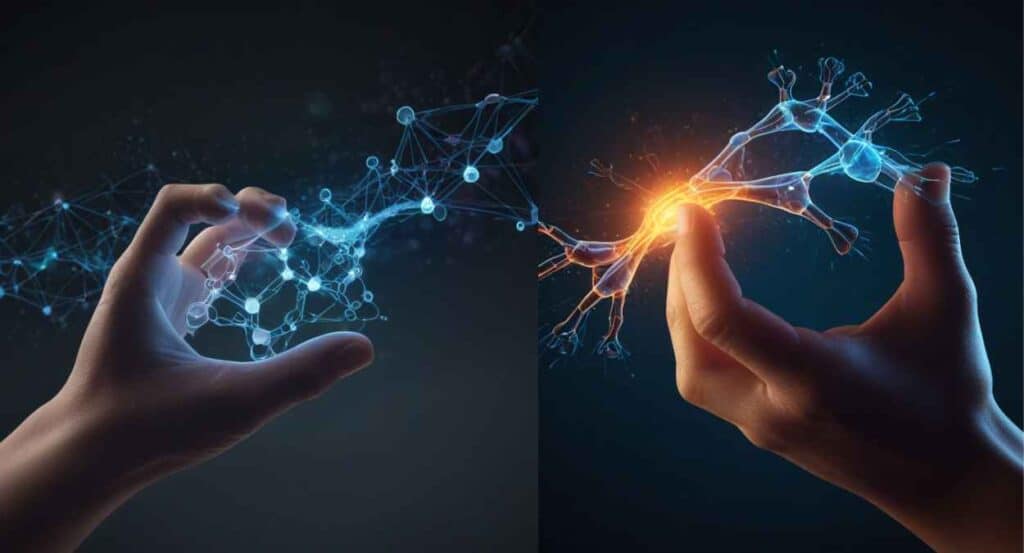
Generative AI is like having a super intelligent assistant that can design entirely new drug molecules from scratch. It learns from massive databases of existing drugs and their effects to create novel compounds that could potentially treat diseases better than current medicines. This amazing AI can explore unlimited new drug possibilities that human scientists may never think of!
What is the Role of Generative AI in Drug Discovery?
The best part is generative AI works incredibly fast compared to traditional drug discovery methods. While finding a new drug traditionally takes 10-15 years, this AI could do it in a fraction of the time. It can quickly test millions of virtual drug designs before homing in on the most promising real candidates.
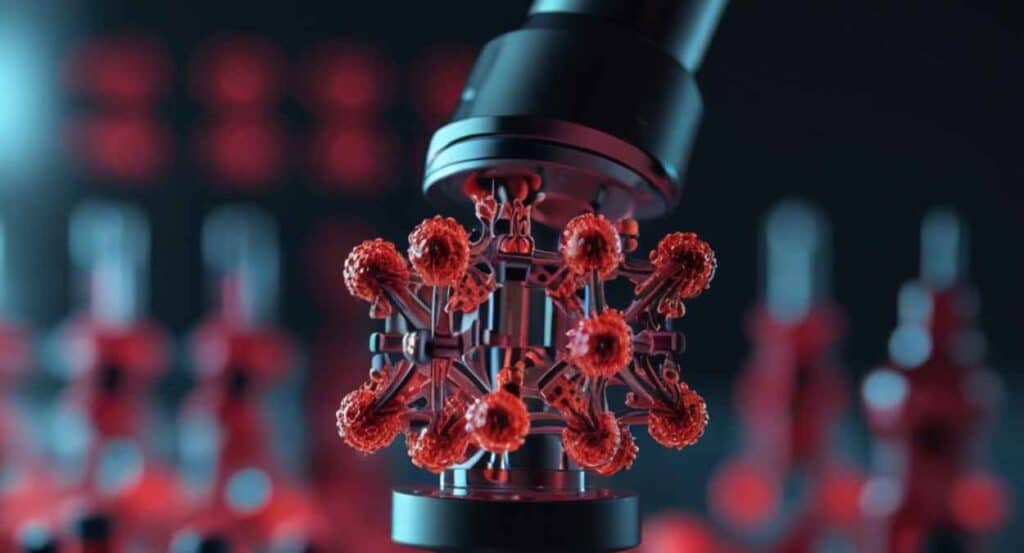
But generative AI doesn’t just design new drugs faster – it can also optimize existing ones to make them more effective, safer, and personalized for each patient’s genetics. It has the potential to create custom-tailored medicines that minimize side effects.
With such a powerful tool, pharmaceutical companies are rushing to implement generative AI. It could lead to groundbreaking new treatments for various illnesses like cancer, rare diseases, antibiotic resistance and more. The future of medicine is looking brilliant thanks to this revolutionary AI technology!
Understanding Generative AI and Its Applications
Generative AI models are a class of algorithms that can create new data, such as images, text, or molecular structures, based on the patterns and relationships learned from existing data. These models leverage techniques like Generative Adversarial Networks (GANs), Variational Autoencoders (VAEs), and diffusion models to generate novel outputs.
In the context of drug discovery, generative AI models can be trained on vast databases of molecular structures and their associated properties, enabling them to generate entirely new drug-like molecules with desired characteristics. This capability opens up exciting possibilities for exploring uncharted chemical spaces and discovering innovative therapeutic solutions.
Benefits of Generative AI over Traditional Methods in Drug Discovery:
- Accelerated Discovery Process: Traditional drug discovery methods can be time-consuming and resource-intensive, often taking years to identify and validate potential drug candidates. Generative AI models can significantly accelerate this process by rapidly generating and optimizing novel molecular structures.
- Expanded Chemical Space: Generative AI algorithms can explore chemical spaces far beyond those covered by existing molecular databases, allowing researchers to uncover novel compounds with unique properties and potentially superior therapeutic effects.
- Data Augmentation: In scenarios where experimental data is limited, generative AI models can leverage existing data to generate synthetic data points, enhancing the training process and improving the accuracy of predictive models.
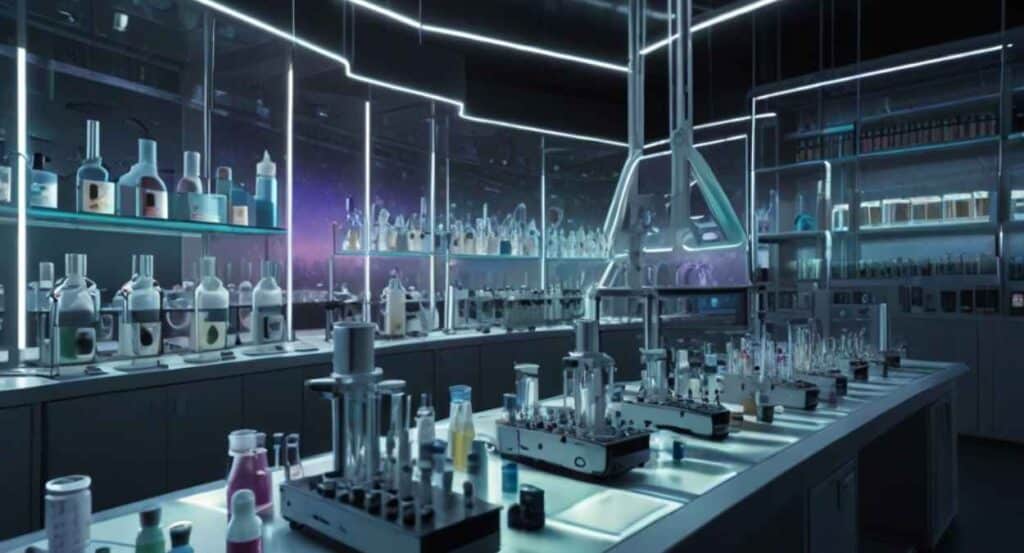
The Drug Discovery Process and Its Challenges
The traditional drug discovery pipeline involves several stages, including target identification, lead discovery, lead optimization, preclinical testing, and clinical trials. Each stage presents its own set of challenges, contributing to the high failure rates and prolonged timelines associated with drug development.
Challenges in the Drug Discovery Process:
- High Costs and Long Timelines: Developing a new drug can cost billions of dollars and take over a decade, making the process highly resource-intensive and financially risky.
- Limited Chemical Space Exploration: Traditional methods often rely on screening existing compound libraries, limiting the exploration of novel chemical spaces and potentially missing out on more effective drug candidates.
- Inefficient Target Identification and Validation: Identifying and validating new drug targets can be a challenging and time-consuming process, hindering the development of treatments for various diseases.
- Suboptimal Lead Optimization: Optimizing lead compounds to improve their pharmacological properties, such as potency, selectivity, and bioavailability, can be a complex and iterative process, leading to delays and increased costs.
- Toxicity and Adverse Effects: Predicting and mitigating the potential toxicity and adverse effects of drug candidates is crucial but can be challenging with traditional methods, leading to costly failures in later stages of development.
By addressing these challenges, generative AI offers a promising solution to accelerate and streamline the drug discovery process, ultimately reducing costs and increasing the likelihood of success.
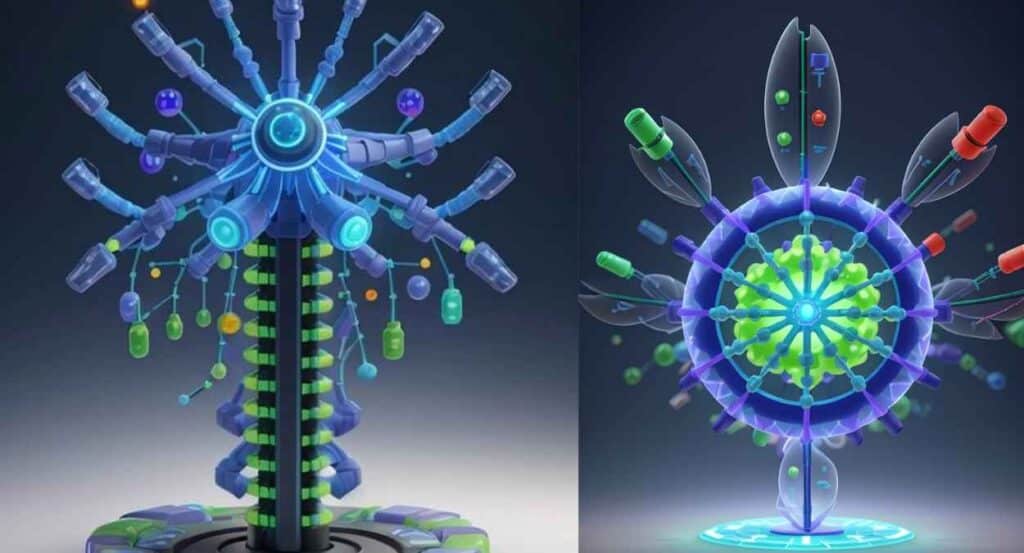
Generative AI in Drug Design and Lead Optimization
One of the primary applications of generative AI in drug discovery is in the design and optimization of new drug candidates. Generative models can be trained on vast databases of molecular structures and their associated properties, enabling them to generate novel compounds with desired characteristics.
De Novo Molecular Design:
Generative AI algorithms can facilitate de novo molecular design, where entirely new chemical structures are created from scratch. This approach allows researchers to explore uncharted chemical spaces and potentially discover compounds with superior therapeutic effects or improved safety profiles.
Lead Optimization and QSAR Modeling:
Once initial lead compounds are identified, generative AI can assist in the optimization process by fine-tuning their molecular structures to enhance desired properties, such as potency, selectivity, or bioavailability. This is achieved through iterative processes that leverage techniques like reinforcement learning and quantitative structure-activity relationship (QSAR) modeling.
Examples and Case Studies:
- Insilico Medicine, a pioneering company in AI-driven drug discovery, used generative AI models to design novel drug-like molecules for various therapeutic areas, including cancer and fibrosis.
- Researchers at the Massachusetts Institute of Technology (MIT) employed generative AI to design novel antibiotics capable of overcoming antibiotic resistance.
- Exscientia, a prominent AI drug discovery company, utilized generative AI to design and optimize novel drug candidates, leading to the discovery of a potential treatment for OCD that entered clinical trials in record time.

Generative AI in Target Identification and Validation
Before the drug design process can begin, researchers must identify and validate potential drug targets – the biological molecules or pathways that a drug aims to modulate or interact with. Generative AI models can play a crucial role in this stage by leveraging techniques like protein structure prediction and disease mechanism modeling.
Protein Structure Prediction:
Generative AI algorithms can be trained to predict the three-dimensional structures of proteins based on their amino acid sequences. This capability is invaluable in understanding the relationship between protein structure and function, enabling researchers to identify potential drug targets and design compounds that can effectively interact with them.
Disease Mechanism Modeling:
By analyzing vast amounts of biomedical data, generative AI models can unravel the complex mechanisms underlying various diseases. This knowledge can inform the identification of novel drug targets and the development of targeted therapies tailored to specific disease pathways.
Example:
- DeepMind, a subsidiary of Alphabet Inc. (Google’s parent company), used its AlphaFold generative AI system to predict the structures of nearly all known proteins, providing invaluable insights for drug target identification and drug design.
Generative AI in Preclinical and Clinical Trials
Generative AI can contribute to the drug discovery process beyond the initial stages of target identification and lead optimization. Its applications extend to preclinical testing, clinical trial design, and the prediction of potential adverse effects.
Preclinical Testing and Animal Studies:
Generative AI models can simulate and optimize preclinical testing procedures, reducing the need for extensive animal studies and accelerating the transition to human clinical trials. These models can predict the efficacy, toxicity, and pharmacokinetic properties of drug candidates, enabling researchers to prioritize the most promising compounds for further evaluation.
Clinical Trial Design and Patient Selection:
By analyzing vast amounts of patient data and historical clinical trial information, generative AI algorithms can optimize the design of clinical trials, ensuring they are tailored to the specific characteristics of the target patient population. This can improve patient recruitment, enhance trial efficiency, and increase the chances of successful outcomes.
Predicting Drug Toxicity and Adverse Effects:
One of the significant challenges in drug development is predicting and mitigating potential toxicity and adverse effects. Generative AI models can be trained on existing data to identify potential safety concerns early in the development process, reducing the risk of costly failures in later stages.
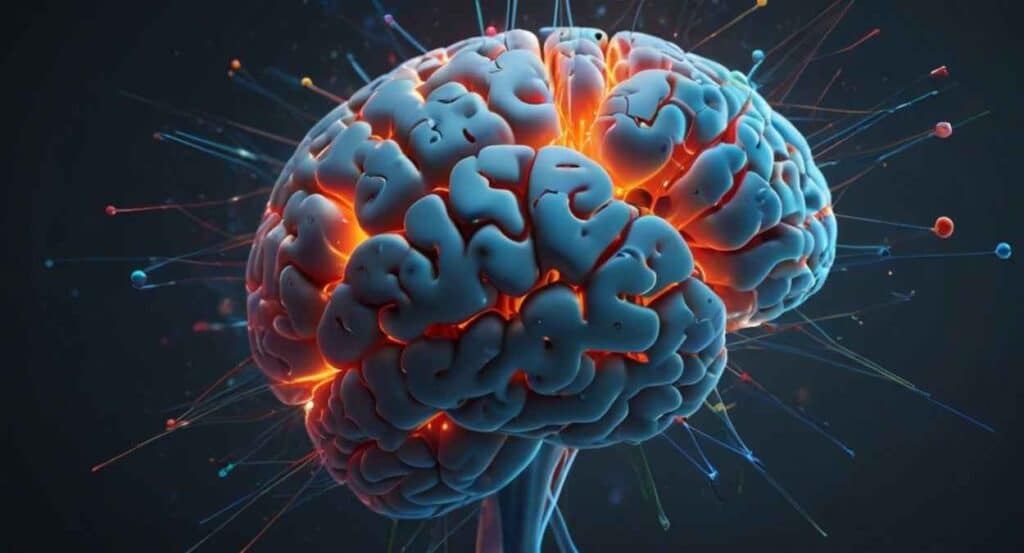
Impact of Generative AI Systems on Drug Discovery
The integration of generative AI into the drug discovery process offers numerous advantages and has the potential to revolutionize the way new drugs are developed:
- Boosting Efficiency and Speed: By automating various stages of the drug discovery pipeline, generative AI can significantly accelerate the process, reducing the time and resources required to identify and validate potential drug candidates.
- Expanding the Chemical Space: Generative AI models can explore chemical spaces far beyond those covered by existing molecular databases, enabling the discovery of novel compounds with unique properties and potentially superior therapeutic effects.
- Targeting Specific Diseases: By leveraging disease mechanism modeling and protein structure prediction, generative AI can facilitate the identification of novel drug targets and the development of treatments tailored to specific diseases or patient populations.
- Overcoming Data Limitations: In scenarios where experimental data is limited, generative AI models can augment existing data by generating synthetic data points, enhancing the training process and improving the accuracy of predictive models.
- Facilitating Collaboration: Generative AI platforms can foster collaboration among researchers by enabling multi-turn conversations and facilitating the exchange of ideas and collective efforts towards solving complex challenges in drug discovery.
Overcoming Drug Resistance
One of the significant challenges in the healthcare industry is the emergence of drug-resistant strains of pathogens, such as antibiotic-resistant bacteria. Generative AI offers potential solutions to combat this issue by designing novel compounds that can target drug-resistant mechanisms or pathways.
Antibiotic Resistance:
The rise of antibiotic-resistant bacteria poses a severe threat to global public health, making it crucial to develop new antibiotics with novel mechanisms of action. Generative AI can aid in the design of antibiotics that can overcome resistance by targeting previously unexplored bacterial targets or pathways.
Example:
- Researchers at MIT used a generative AI model to design novel antibiotic compounds capable of overcoming resistance mechanisms in bacteria like E. coli and Acinetobacter baumannii.
Targeting Drug Resistance in Cancer:
Drug resistance is also a significant challenge in cancer treatment, as tumors can develop resistance to chemotherapeutic agents over time. Generative AI can be employed to design novel compounds that can overcome these resistance mechanisms, potentially improving treatment outcomes for cancer patients.
Offering Personalized Therapies
One of the most promising applications of generative AI in drug discovery is the development of personalized therapies tailored to individual patients or specific disease subtypes. By leveraging patient-specific data, such as genetic information, lifestyle factors, and disease characteristics, generative AI algorithms can be programmed to focus on specific disease targets or mechanisms.
Precision Medicine:
Generative AI can aid in the development of precision medicine approaches by designing drug candidates that consider an individual’s genetic makeup, molecular profiles, and other relevant factors. This personalized approach has the potential to improve treatment efficacy and reduce the risk of adverse effects.
Example:
- Insitro, a biotechnology company, is leveraging generative AI to develop personalized therapies for specific disease subtypes based on multi-omics data and patient-specific characteristics.
Challenges and Limitations
While generative AI holds immense potential in revolutionizing drug discovery, it is crucial to acknowledge and address the challenges and limitations associated with its implementation:
- Interpretability and Trustworthiness: Generative AI models, particularly deep learning algorithms, can often be perceived as “black boxes,” making it challenging to interpret and understand their decision-making processes. This lack of interpretability can raise concerns regarding the trustworthiness of the generated outputs, especially in the context of drug discovery, where safety and efficacy are paramount.
- Potential Biases and Ethical Concerns: Like any AI system, generative AI models can be susceptible to biases present in the training data or introduced during the model development process. These biases can lead to undesirable outcomes or perpetuate existing disparities. Additionally, there are ethical considerations surrounding the generation of potentially harmful or uncontrolled outputs, which need to be carefully addressed.
- Need for Robust Validation and Testing: While generative AI can accelerate the discovery process, the drug candidates generated by these models still require rigorous experimental validation and testing to ensure their safety, efficacy, and compliance with regulatory requirements. Comprehensive validation protocols need to be established to mitigate potential risks.
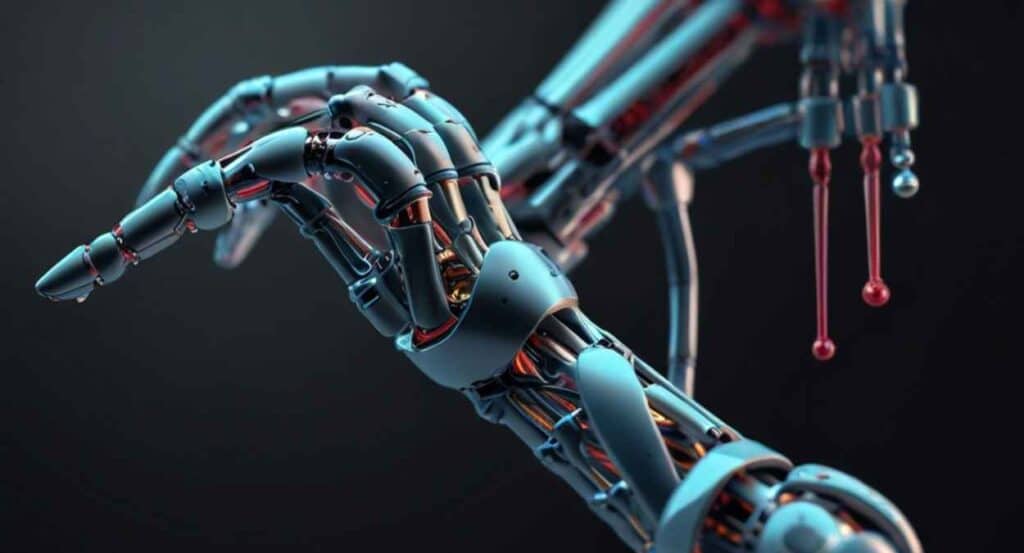
Future Implications and Trends
The integration of generative AI into drug discovery is just the beginning of a transformative journey. As this technology continues to evolve and mature, it holds the potential to reshape the entire pharmaceutical industry and pave the way for groundbreaking advancements:
- Personalized Medicine: Generative AI will play a crucial role in realizing the vision of personalized medicine, where treatments are tailored to an individual’s unique genetic makeup, lifestyle factors, and disease characteristics. This approach has the potential to significantly improve treatment outcomes and minimize adverse effects.
- Rare Disease Treatments: By exploring novel chemical spaces and leveraging disease mechanism modeling, generative AI can facilitate the discovery of potential treatments for rare diseases that have traditionally been overlooked due to limited financial incentives and small patient populations.
- Drug Repurposing: Generative AI can aid in the identification of new uses for existing drugs by analyzing their mechanisms of action and potential interactions with different biological targets. This approach can expedite the development of treatments for new indications, reducing the time and costs associated with traditional drug discovery pipelines.
- Integration with Other Emerging Technologies: Generative AI will likely be combined with other cutting-edge technologies, such as quantum computing, synthetic biology, and organ-on-a-chip models, to create powerful synergies and further accelerate drug discovery and development processes.
- Interdisciplinary Collaboration: The successful implementation of generative AI in drug discovery will require close collaboration between AI experts, drug researchers, clinicians, and regulatory authorities. This interdisciplinary approach will foster a deeper understanding of the technology’s potential and ensure its responsible and ethical development.
As the field of generative AI continues to evolve, it is essential for stakeholders across the pharmaceutical industry to stay informed about the latest developments and actively participate in shaping the future of drug discovery.
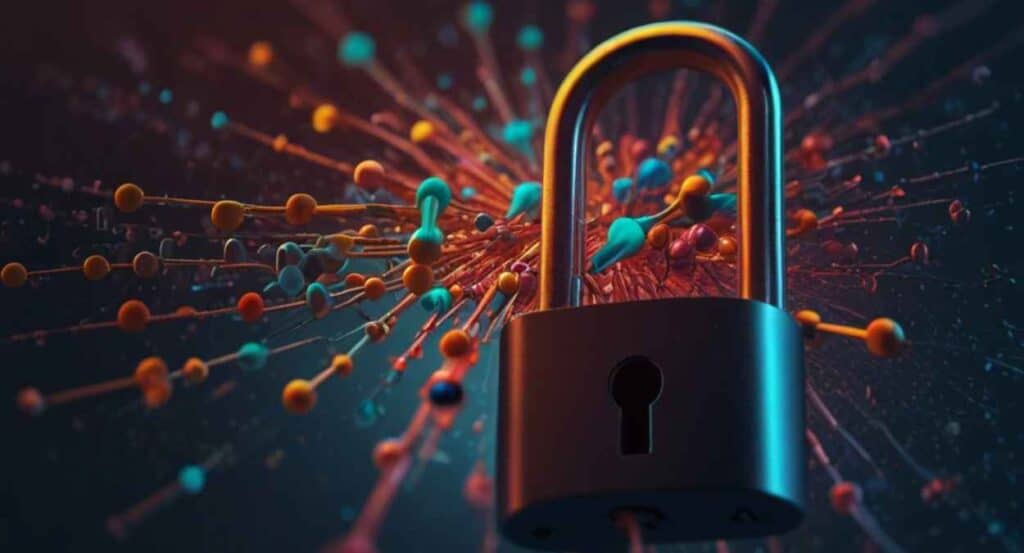
Frequently Asked Questions (FAQs)
Q. How does generative AI differ from traditional AI methods in drug discovery?
A. Generative AI models can create entirely new data, such as novel molecular structures, based on patterns learned from existing data. This capability expands the exploration of chemical spaces beyond traditional methods, which often rely on screening existing compound libraries. Generative AI also enables the generation of synthetic data to augment limited experimental datasets.
Q. What are some common generative AI models used in drug discovery?
A. Popular generative AI models used in drug discovery include Generative Adversarial Networks (GANs), Variational Autoencoders (VAEs), and diffusion models. These models can generate novel drug-like molecules, predict protein structures, and model disease mechanisms.
Q. How can generative AI contribute to personalized medicine?
A. Generative AI can aid in the development of personalized therapies by designing drug candidates tailored to individual patients’ genetic makeup, molecular profiles, and disease characteristics. This personalized approach has the potential to improve treatment efficacy and reduce the risk of adverse effects.
Q. What are the challenges associated with implementing generative AI in drug discovery?
A. Key challenges include ensuring the interpretability and trustworthiness of generative AI models, addressing potential biases and ethical concerns, and establishing robust validation protocols for AI-generated drug candidates.
Q. How can generative AI assist in overcoming drug resistance?
A. Generative AI can be used to design novel compounds that target previously unexplored pathways or mechanisms, enabling the development of new antibiotics and cancer treatments that can overcome drug resistance.
Q. What is the role of generative AI in rare disease treatments?
A. By exploring novel chemical spaces and leveraging disease mechanism modeling, generative AI can facilitate the discovery of potential treatments for rare diseases that have traditionally been overlooked due to limited financial incentives and small patient populations.
Q. How can generative AI contribute to drug repurposing?
A. Generative AI models can analyze the mechanisms of action and potential interactions of existing drugs with different biological targets, enabling the identification of new therapeutic applications for these drugs, thereby accelerating the development of treatments for new indications.
Watch the Web story on ‘What is the role of Generative AI in Drug Discovery ?’
Conclusion
Hope you have understood about What is the role of Generative AI in Drug Discovery ? The integration of generative AI into the drug discovery process represents a paradigm shift in the way new drugs are developed. By leveraging advanced algorithms and vast data repositories, generative AI models can accelerate the identification and optimization of novel drug candidates, expand the exploration of chemical spaces, and facilitate the development of personalized therapies tailored to individual patients.
As the pharmaceutical industry continues to grapple with the challenges of lengthy development timelines, high costs, and the constant threat of drug resistance, generative AI emerges as a powerful tool to address these issues. Its applications span various stages of the drug discovery pipeline, from target identification and lead optimization to preclinical testing and clinical trial design.
However, the successful implementation of generative AI in drug discovery requires addressing challenges such as interpretability, potential biases, and the need for robust validation protocols. It is crucial to establish a strong foundation of trust and transparency, ensuring that the generated outputs are reliable, safe, and compliant with regulatory requirements.
As we look to the future, the integration of generative AI with other emerging technologies, such as quantum computing and synthetic biology, holds the promise of further accelerating drug discovery and development processes. Additionally, the interdisciplinary collaboration between AI experts, drug researchers, clinicians, and regulatory authorities will be essential in shaping the responsible and ethical development of this transformative technology.
The potential impact of generative AI in drug discovery extends far beyond the pharmaceutical industry itself. By unlocking new frontiers in personalized medicine, rare disease treatments, and drug repurposing, this technology holds the power to revolutionize healthcare and improve the lives of millions of people worldwide.
Disclaimer
The information provided on AI Wini’s blog regarding generative AI in drug discovery is intended for educational and informational purposes only and should not be construed as professional medical advice.
Also Read :
Revolutionizing Mobile AI: iPhone 16’s Game-Changing On-Device Generative AI
Meta AI Chatbot Rolls Out on Instagram, WhatsApp & Messenger – The New Era Begins!
Microsoft & OpenAI’s $100B Stargate AI Supercomputer: How This Supercomputer Will Change Everything?